Are You Building AI Products That Truly Resonate With Your Users?
Are You Building AI Products That Truly Resonate With Your Users?
By
Mark Whaley
on •
Sep 25, 2024
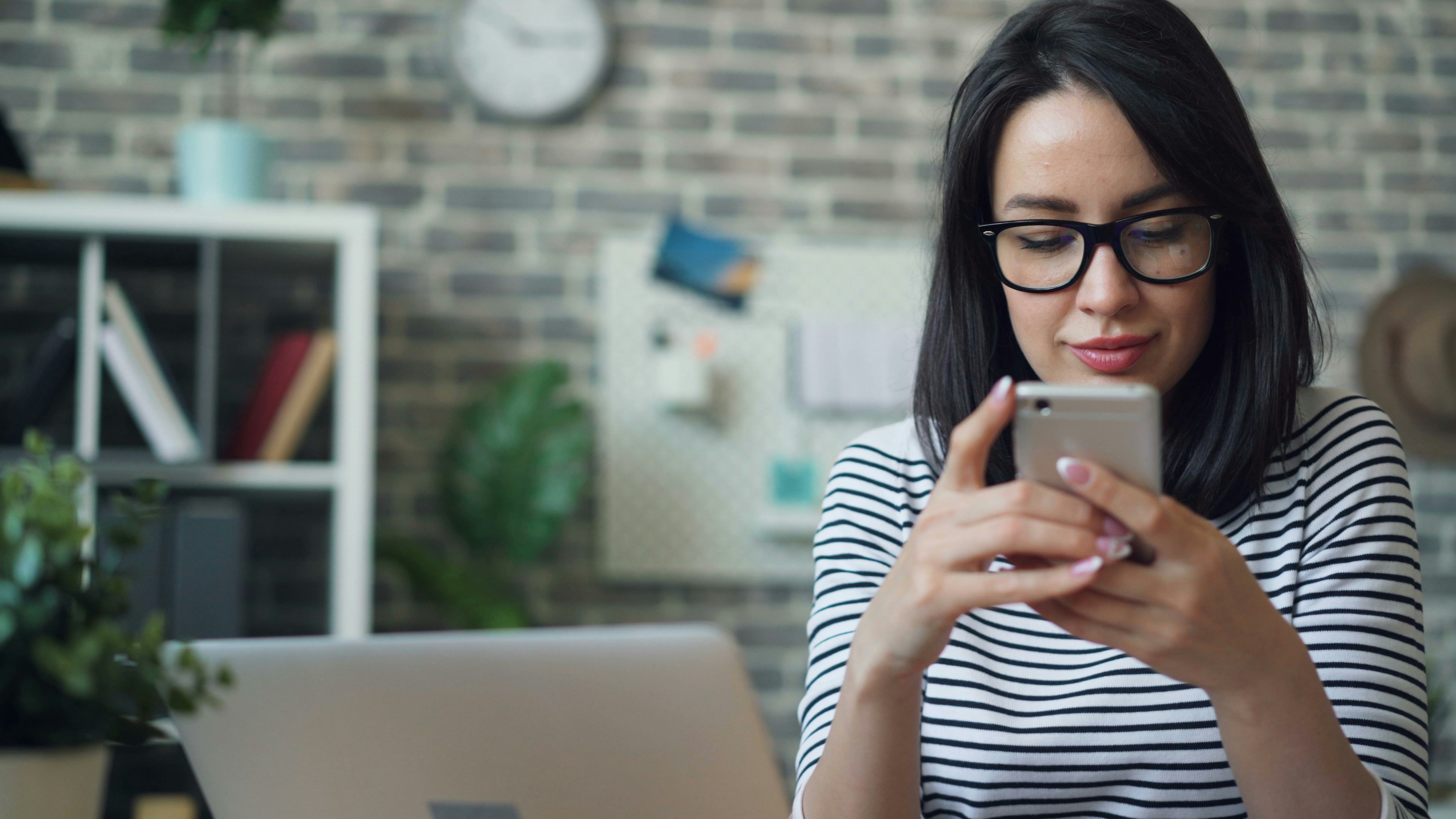
AI is set to revolutionize many aspects of software products and development. However, one thing that has not changed is the fundamentals of Product-Market Fit. You still need to identify an opportunity that genuinely matters to users, develop a strategic product roadmap, and iteratively deliver something they can't live without.
Amid the current AI hype, it's common to see companies rush into developing innovative AI products without fully understanding what AI is capable of doing or taking the essential steps to establish customer alignment.
In this post, Mark Whaley, Head of Product at Artium, shares his insights on building AI products that are not just viable but truly valuable.
What are the biggest challenges in defining a viable AI product roadmap?
With any roadmap (not just AI), the biggest challenge I see is the misalignment between business goals and how to implement them—clarifying the solution you want to build and figuring out the best way to build it.
One common pitfall, especially with AI, is the gap between what people read in the news and what's actually feasible and helpful for a business. Business owners sometimes get fixated on the latest tech trends, like large language models (LLMs), when they could be solving that same problem rather simply with a traditional tech build. If you're paying attention to what the user really needs then oftentimes you may not even need AI.
The other piece that I want to highlight is that even if AI might be a good solution for what you want to build, you may not have the knowledge or resources to build it properly. For example, there are a number of different AI architectures that can be implemented for different use cases. Do you want a simple AI chat, are you transforming data with AI assistance, or are you going for a more complex multi-model generative interface? Many businesses don’t realize the magnitude of these fundamental decisions.
In short, to get a clear roadmap you have to take into account the capabilities of AI combined with tangible business goals, the infrastructure and data in place to back it up.
How do you balance innovation with practicality when selecting AI projects to pursue?
I will always prioritize practical applications with a clear path towards deployment and a measurable business impact. I think that a lot of times leaders find themselves hooked by the allure of a novel tech advancement, but they may run into practical constraints like time, money, and customer needs. Innovation is exciting, but if you chase it for its own sake, you can end up with solutions that are too complex or don’t really solve the customer’s problem.
My advice is to always start with an AI project that addresses well-defined customer pain points and delivers measurable outcomes. This will allow you to set realistic timelines and investment thresholds. For example, choosing to build an AI solution for optimizing appointment scheduling might be less glamorous than something like a cutting-edge disease diagnosis system, but it’s much more practical and can deliver immediate business value.
When coming up with a new implementation for AI, I’d suggest you start small, solve a tangible problem, then build from there. Once you have success with a smaller, practical solution, you can expand into more ambitious projects.
What strategies do you use to validate that an AI solution is truly adding value to the customer?
Again, a good product manager focuses on solving specific, well-defined issues. This means making sure the AI solution is tied directly to real-world customer needs.
You validate by collecting both quantitative and qualitative feedback as you build. You want to measure AI's impact on efficiency, user experience, and revenue impact. Data drives your decisions, and that data should come from both customer interviews and how people are actually interacting with the product.
For example, if you make a change in a prompt that you're writing for a LLM, you would want to immediately measure what that's doing to your customer experience and its impact on how they're moving through your product. Use frequent iterations where the models are tested then refined based on that measurement.
Take a chatbot as an example. It may be technically impressive but it may still receive poor feedback from users. Chatbots can be challenging to talk to, hard to navigate or not really helpful for specific queries. Users end up getting fed up with them and just go to a website FAQ or ask to talk to a real person. To fix this, companies can refine their chatbot experience by adding in natural language processing capabilities and train the model using real customer data. Through that process the experience will get better over time.
Adopting a “customer mindset” is something everyone talks about, but hardly anyone really does. I’ve seen this in larger organizations that claim to be customer-centric but don’t actually conduct interviews or measure the impact of their decisions on real users. AI-driven products need continuous refinement, so it’s critical to make user feedback and continuous discovery a part of your development lifecycle.
How do you integrate user feedback into the development process for AI-driven products? What key metrics do you use to measure the success of AI initiatives?
The key is to involve users early and often. Many teams make the mistake of building first and asking for feedback later, but we prefer to integrate user feedback right from the ideation phase. That way, the product evolves with the customer in mind from the very beginning.
We use beta testing, pilot programs, early prompt testing, and iterative development cycles to refine our solutions based on real-world feedback loops. Metrics like customer satisfaction, AI model accuracy, and business performance are vital. But some metrics are more intuitive—like whether a chatbot feels human, which can drive engagement.
What role does cross-functional collaboration play in the success of AI projects, and how do you foster this approach at Artium?
Cross-functional collaboration is the cornerstone of what we do at Artium. AI projects are inherently multidisciplinary, requiring input from engineers, AI architects, product managers, designers, and business stakeholders. You need this blend of perspectives to ensure a holistic approach to building AI solutions.
At Artium, we emphasize the importance of frequent, clear communication across teams. We don’t throw a roadmap over the fence for the engineering team to execute; instead, we work together continuously. Regular touchpoints, shared artifacts, and a culture of transparency ensure everyone stays aligned.
For example, if we’re developing an AI-powered recommendation feature for a mobile app, it’s not just the engineers focusing on the technical aspects. Designers ensure the user experience is seamless, AI architects ensure reliability, alignment, and accuracy, and the product managers ensure business goals are met. If any one of these elements are out of sync, the project will fail to meet user expectations.
We also hold weekly retrospectives to adapt based on feedback and ensure we’re always focused on delivering rapid tangible outcomes.
AI is set to revolutionize many aspects of software products and development. However, one thing that has not changed is the fundamentals of Product-Market Fit. You still need to identify an opportunity that genuinely matters to users, develop a strategic product roadmap, and iteratively deliver something they can't live without.
Amid the current AI hype, it's common to see companies rush into developing innovative AI products without fully understanding what AI is capable of doing or taking the essential steps to establish customer alignment.
In this post, Mark Whaley, Head of Product at Artium, shares his insights on building AI products that are not just viable but truly valuable.
What are the biggest challenges in defining a viable AI product roadmap?
With any roadmap (not just AI), the biggest challenge I see is the misalignment between business goals and how to implement them—clarifying the solution you want to build and figuring out the best way to build it.
One common pitfall, especially with AI, is the gap between what people read in the news and what's actually feasible and helpful for a business. Business owners sometimes get fixated on the latest tech trends, like large language models (LLMs), when they could be solving that same problem rather simply with a traditional tech build. If you're paying attention to what the user really needs then oftentimes you may not even need AI.
The other piece that I want to highlight is that even if AI might be a good solution for what you want to build, you may not have the knowledge or resources to build it properly. For example, there are a number of different AI architectures that can be implemented for different use cases. Do you want a simple AI chat, are you transforming data with AI assistance, or are you going for a more complex multi-model generative interface? Many businesses don’t realize the magnitude of these fundamental decisions.
In short, to get a clear roadmap you have to take into account the capabilities of AI combined with tangible business goals, the infrastructure and data in place to back it up.
How do you balance innovation with practicality when selecting AI projects to pursue?
I will always prioritize practical applications with a clear path towards deployment and a measurable business impact. I think that a lot of times leaders find themselves hooked by the allure of a novel tech advancement, but they may run into practical constraints like time, money, and customer needs. Innovation is exciting, but if you chase it for its own sake, you can end up with solutions that are too complex or don’t really solve the customer’s problem.
My advice is to always start with an AI project that addresses well-defined customer pain points and delivers measurable outcomes. This will allow you to set realistic timelines and investment thresholds. For example, choosing to build an AI solution for optimizing appointment scheduling might be less glamorous than something like a cutting-edge disease diagnosis system, but it’s much more practical and can deliver immediate business value.
When coming up with a new implementation for AI, I’d suggest you start small, solve a tangible problem, then build from there. Once you have success with a smaller, practical solution, you can expand into more ambitious projects.
What strategies do you use to validate that an AI solution is truly adding value to the customer?
Again, a good product manager focuses on solving specific, well-defined issues. This means making sure the AI solution is tied directly to real-world customer needs.
You validate by collecting both quantitative and qualitative feedback as you build. You want to measure AI's impact on efficiency, user experience, and revenue impact. Data drives your decisions, and that data should come from both customer interviews and how people are actually interacting with the product.
For example, if you make a change in a prompt that you're writing for a LLM, you would want to immediately measure what that's doing to your customer experience and its impact on how they're moving through your product. Use frequent iterations where the models are tested then refined based on that measurement.
Take a chatbot as an example. It may be technically impressive but it may still receive poor feedback from users. Chatbots can be challenging to talk to, hard to navigate or not really helpful for specific queries. Users end up getting fed up with them and just go to a website FAQ or ask to talk to a real person. To fix this, companies can refine their chatbot experience by adding in natural language processing capabilities and train the model using real customer data. Through that process the experience will get better over time.
Adopting a “customer mindset” is something everyone talks about, but hardly anyone really does. I’ve seen this in larger organizations that claim to be customer-centric but don’t actually conduct interviews or measure the impact of their decisions on real users. AI-driven products need continuous refinement, so it’s critical to make user feedback and continuous discovery a part of your development lifecycle.
How do you integrate user feedback into the development process for AI-driven products? What key metrics do you use to measure the success of AI initiatives?
The key is to involve users early and often. Many teams make the mistake of building first and asking for feedback later, but we prefer to integrate user feedback right from the ideation phase. That way, the product evolves with the customer in mind from the very beginning.
We use beta testing, pilot programs, early prompt testing, and iterative development cycles to refine our solutions based on real-world feedback loops. Metrics like customer satisfaction, AI model accuracy, and business performance are vital. But some metrics are more intuitive—like whether a chatbot feels human, which can drive engagement.
What role does cross-functional collaboration play in the success of AI projects, and how do you foster this approach at Artium?
Cross-functional collaboration is the cornerstone of what we do at Artium. AI projects are inherently multidisciplinary, requiring input from engineers, AI architects, product managers, designers, and business stakeholders. You need this blend of perspectives to ensure a holistic approach to building AI solutions.
At Artium, we emphasize the importance of frequent, clear communication across teams. We don’t throw a roadmap over the fence for the engineering team to execute; instead, we work together continuously. Regular touchpoints, shared artifacts, and a culture of transparency ensure everyone stays aligned.
For example, if we’re developing an AI-powered recommendation feature for a mobile app, it’s not just the engineers focusing on the technical aspects. Designers ensure the user experience is seamless, AI architects ensure reliability, alignment, and accuracy, and the product managers ensure business goals are met. If any one of these elements are out of sync, the project will fail to meet user expectations.
We also hold weekly retrospectives to adapt based on feedback and ensure we’re always focused on delivering rapid tangible outcomes.
AI is set to revolutionize many aspects of software products and development. However, one thing that has not changed is the fundamentals of Product-Market Fit. You still need to identify an opportunity that genuinely matters to users, develop a strategic product roadmap, and iteratively deliver something they can't live without.
Amid the current AI hype, it's common to see companies rush into developing innovative AI products without fully understanding what AI is capable of doing or taking the essential steps to establish customer alignment.
In this post, Mark Whaley, Head of Product at Artium, shares his insights on building AI products that are not just viable but truly valuable.
What are the biggest challenges in defining a viable AI product roadmap?
With any roadmap (not just AI), the biggest challenge I see is the misalignment between business goals and how to implement them—clarifying the solution you want to build and figuring out the best way to build it.
One common pitfall, especially with AI, is the gap between what people read in the news and what's actually feasible and helpful for a business. Business owners sometimes get fixated on the latest tech trends, like large language models (LLMs), when they could be solving that same problem rather simply with a traditional tech build. If you're paying attention to what the user really needs then oftentimes you may not even need AI.
The other piece that I want to highlight is that even if AI might be a good solution for what you want to build, you may not have the knowledge or resources to build it properly. For example, there are a number of different AI architectures that can be implemented for different use cases. Do you want a simple AI chat, are you transforming data with AI assistance, or are you going for a more complex multi-model generative interface? Many businesses don’t realize the magnitude of these fundamental decisions.
In short, to get a clear roadmap you have to take into account the capabilities of AI combined with tangible business goals, the infrastructure and data in place to back it up.
How do you balance innovation with practicality when selecting AI projects to pursue?
I will always prioritize practical applications with a clear path towards deployment and a measurable business impact. I think that a lot of times leaders find themselves hooked by the allure of a novel tech advancement, but they may run into practical constraints like time, money, and customer needs. Innovation is exciting, but if you chase it for its own sake, you can end up with solutions that are too complex or don’t really solve the customer’s problem.
My advice is to always start with an AI project that addresses well-defined customer pain points and delivers measurable outcomes. This will allow you to set realistic timelines and investment thresholds. For example, choosing to build an AI solution for optimizing appointment scheduling might be less glamorous than something like a cutting-edge disease diagnosis system, but it’s much more practical and can deliver immediate business value.
When coming up with a new implementation for AI, I’d suggest you start small, solve a tangible problem, then build from there. Once you have success with a smaller, practical solution, you can expand into more ambitious projects.
What strategies do you use to validate that an AI solution is truly adding value to the customer?
Again, a good product manager focuses on solving specific, well-defined issues. This means making sure the AI solution is tied directly to real-world customer needs.
You validate by collecting both quantitative and qualitative feedback as you build. You want to measure AI's impact on efficiency, user experience, and revenue impact. Data drives your decisions, and that data should come from both customer interviews and how people are actually interacting with the product.
For example, if you make a change in a prompt that you're writing for a LLM, you would want to immediately measure what that's doing to your customer experience and its impact on how they're moving through your product. Use frequent iterations where the models are tested then refined based on that measurement.
Take a chatbot as an example. It may be technically impressive but it may still receive poor feedback from users. Chatbots can be challenging to talk to, hard to navigate or not really helpful for specific queries. Users end up getting fed up with them and just go to a website FAQ or ask to talk to a real person. To fix this, companies can refine their chatbot experience by adding in natural language processing capabilities and train the model using real customer data. Through that process the experience will get better over time.
Adopting a “customer mindset” is something everyone talks about, but hardly anyone really does. I’ve seen this in larger organizations that claim to be customer-centric but don’t actually conduct interviews or measure the impact of their decisions on real users. AI-driven products need continuous refinement, so it’s critical to make user feedback and continuous discovery a part of your development lifecycle.
How do you integrate user feedback into the development process for AI-driven products? What key metrics do you use to measure the success of AI initiatives?
The key is to involve users early and often. Many teams make the mistake of building first and asking for feedback later, but we prefer to integrate user feedback right from the ideation phase. That way, the product evolves with the customer in mind from the very beginning.
We use beta testing, pilot programs, early prompt testing, and iterative development cycles to refine our solutions based on real-world feedback loops. Metrics like customer satisfaction, AI model accuracy, and business performance are vital. But some metrics are more intuitive—like whether a chatbot feels human, which can drive engagement.
What role does cross-functional collaboration play in the success of AI projects, and how do you foster this approach at Artium?
Cross-functional collaboration is the cornerstone of what we do at Artium. AI projects are inherently multidisciplinary, requiring input from engineers, AI architects, product managers, designers, and business stakeholders. You need this blend of perspectives to ensure a holistic approach to building AI solutions.
At Artium, we emphasize the importance of frequent, clear communication across teams. We don’t throw a roadmap over the fence for the engineering team to execute; instead, we work together continuously. Regular touchpoints, shared artifacts, and a culture of transparency ensure everyone stays aligned.
For example, if we’re developing an AI-powered recommendation feature for a mobile app, it’s not just the engineers focusing on the technical aspects. Designers ensure the user experience is seamless, AI architects ensure reliability, alignment, and accuracy, and the product managers ensure business goals are met. If any one of these elements are out of sync, the project will fail to meet user expectations.
We also hold weekly retrospectives to adapt based on feedback and ensure we’re always focused on delivering rapid tangible outcomes.