Beyond Chat: How AI is Transforming UI Design Patterns
Beyond Chat: How AI is Transforming UI Design Patterns
By
James
on •
May 23, 2025
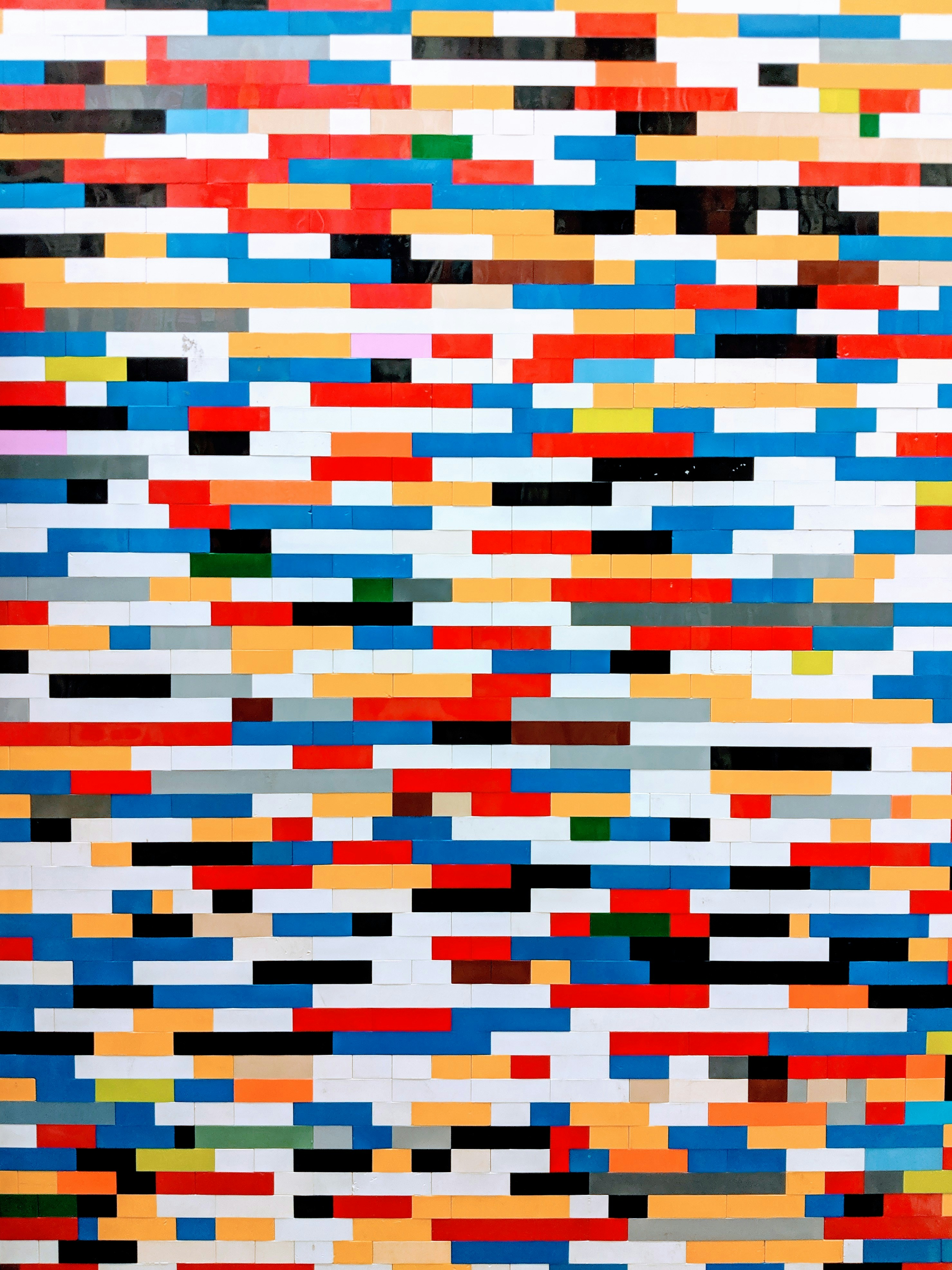
The Chat Disappointment
I initially embraced the chatbot interface like everyone else in the design world. It seemed like this perfect marriage of AI capability and user familiarity - a simple text box where users could ask for anything they needed. When we began our work on an innovation management platform for medical researchers, it felt natural to center the experience around a conversational AI interface.
We built beautiful prototypes. We crafted detailed prompt engineering. We were confident we'd created something revolutionary.
And then we put it in front of actual users.
Chat was very confusing, and actually would dominate the entire experience so much so that people wouldn't look elsewhere on the screen. They were missing a lot of the context that was being created for them. The chat interface wasn't just underperforming—it was actively undermining the experience we wanted to create.
This is the story of how I discovered that the future of AI interfaces lies not in replicating human conversation, but in reimagining UI patterns that create true human-AI collaboration.
The Journey to Something Better
The initial stakeholder excitement about chat interfaces created its own momentum. There's something deeply compelling about the demo of a responsive AI chatbot answering questions with human-like fluency. This excitement led to our POC (proof of concept) being built around a chat-first approach.
But as we continued user testing, the evidence became impossible to ignore. Users weren't effectively accessing the key information and capabilities of the system through chat alone. They struggled to form effective queries and often missed important details that were presented in the chat stream.
We spent a lot of cycles helping our client understand that the POC, at least the user experience side of the POC, we needed to forget about if we wanted to make a good experience for the users. This was a pivotal moment—admitting that despite all the initial excitement, we needed to fundamentally rethink our approach.
Breaking free from the constraints of chat-only interfaces opened up an entirely new world of design possibilities. I began exploring how AI could enhance traditional UI patterns rather than replace them. What if, instead of forcing users to engage with an AI through conversation, we let the AI enhance the interfaces users already understood?
Emerging Patterns That Transformed the Experience
Dynamic Blocks: UI Elements That Respond to Context

Our breakthrough came in the form of what I call "dynamic blocks"—UI components that appear, populate, and adapt based on AI analysis of user needs and context.
For medical innovators uploading documents about their research, the system began analyzing the content and automatically structuring it according to the Stanford Bio Design framework. But rather than presenting this analysis as a chat message, we displayed it as a visual grid of information blocks, each representing a different aspect of the innovation.
The transformation was remarkable. Instead of users having to parse through chat messages to find relevant information, they could immediately see what the AI had understood and what it was missing. The interface became a living, breathing entity that evolved alongside the user's work.
One innovator with over a dozen patents and technologies already in hospital settings told me this thing is already allowing him to spend more time with his family. He actually has a deeper sense of peace, knowing that there's this tool that's doing active research and is aware of market changes in the cases that he's innovating in, and he doesn't have to use so much mental bandwidth to keep track of all of it.
By shifting from chat to dynamic UI elements, we'd created something that felt less like talking to a robot and more like working with an intelligent system that anticipated needs.
Governor Patterns: Building Trust Through Verification
A key insight I gained was understanding the importance of "governor patterns"—UI elements that give users control over AI-generated content. You can read an excellent overview of governor patterns on Shapeof.ai.
Rather than having the AI automatically populate information that might be incorrect or misaligned with the user's intent, we designed a review workflow. When new information blocks appeared, they were initially displayed at 70% opacity to indicate their provisional status. Users could review the content, make adjustments if needed, and then approve it—bringing the block to full opacity and marking it as verified.
This seemingly simple pattern had profound effects on user trust. By acknowledging the imperfect nature of AI and giving users the final say, we transformed AI from a black box into a collaborative tool. The governor pattern created what I'd describe as a human-in-the-loop feedback loop that maintained the user's sense of ownership while still leveraging AI capabilities.
Milestone Markers: Guiding Without Controlling
The third pattern that fundamentally transformed our interface was what I call "milestone markers"—visual indicators that help users understand their progress and suggest potential next steps.
In a traditional interface, the path forward is predetermined by the designer. In a chat interface, the path can feel completely unstructured. Milestone markers represent a middle ground—AI-generated suggestions that guide without restricting.
For our medical innovators, these markers highlighted potential gaps in their innovation documentation and suggested resources to address them. Rather than forcing users down a linear path, the AI analyzed their unique situation and offered personalized guidance.
The user gets a ton of visual affordance to sort of know what's left, what have I done. And it does deliver quite a bit of delight to the user coming in... This system is already telling you where the gaps exist in your idea and really what you need to work on to take it forward. And we do all of this without a chat interaction, without you needing to read through a ton of text or understand how to use AI.
The Designer's Challenge
Creating these new patterns wasn't without significant challenges. Perhaps the biggest was in prototyping and testing.
Traditional prototyping tools like Figma excel at creating static mockups of predetermined states. But how do you prototype an interface that adapts dynamically to each user? How do you test an experience that might be different for every person who uses it?
Each one is like a bit of a snowflake, and the only way that we're able to assess quality and reliability is to get in front of the customer with working software so that they can make that determination for themselves.
This required a fundamental shift in our design process. Rather than creating comprehensive mockups of every possible state, I focused on designing at the atomic level—creating flexible components that could be assembled in countless ways.
We created what I call design components using atomic level design thinking... starting with your smallest parts, packaging up those atomic elements into organisms, blowing those organisms out into templates, sharing those templates with the developers... and then the developers implementing it in a way that ultimately leads to like thousands of variations.
The result was a design system that could scale to accommodate the unpredictable nature of AI interactions while maintaining visual consistency and usability.
For designers accustomed to controlling every pixel, this was a profound shift. In a traditional design world, you would try to design for all of those variants because it was very deterministic. But in this new world, it's not so black and white, it's not so straightforward. It's really taking out all the context that it has and then serving up a user interface that's really personalized individualized for that end user.
Lessons for Designers Creating AI Interfaces
Through this journey of redesigning AI interfaces beyond chat, I've gained several key insights that can guide other designers working on similar challenges:
1. Recognize the limitations of chat-only interfaces
While chat is intuitive and requires minimal onboarding, it often struggles to effectively organize complex information or guide users through multifaceted tasks. Don't let the initial excitement of conversational AI blind you to its limitations.
2. Design for collaboration, not replacement
The most effective AI interfaces don't try to replace traditional UI elements—they enhance them. Think of AI as a collaborative partner that works alongside familiar interface patterns, not a replacement for them.
3. Build governor patterns into your design
Users need to feel in control, especially when working with AI systems. Design explicit approval mechanisms that acknowledge the provisional nature of AI-generated content and give users the final say.
4. Create systems, not screens
With AI-driven interfaces, you're no longer designing static screens—you're designing systems that can generate countless variations. Focus on creating robust design systems with clear rules rather than trying to anticipate every possible state.
5. Develop new prototyping approaches
Traditional prototyping methods fall short for AI interfaces. I wish there was a button in Figma where I could create an AI dynamic track and then dynamically populate the values to create endless variations of the same components. Since that doesn't exist yet, consider creating "scenario prototypes" that show a few realistic variations, then move quickly to working software for true validation.
6. Challenge stakeholder assumptions
The allure of chatbots can create unrealistic expectations among stakeholders. Be prepared to advocate for more effective approaches based on user research, even when it means pushing back against initial excitement.
A New Design Partnership
The future of UI design isn't about choosing between traditional interfaces and AI—it's about forging a new kind of partnership between humans and intelligent systems. The patterns I've explored—dynamic blocks, governor mechanisms, and milestone markers—represent early steps toward interfaces that combine AI capabilities with human-centered design principles.
For our medical innovators, this partnership has been transformative. As one user told me, the system has given him a deeper sense of peace, knowing that there's this tool that's doing active research and is aware of market changes. It's allowed him to focus on what matters most—both in his work and in his life.
As I continue exploring this new frontier of design, the goal remains the same: creating experiences that amplify human capabilities rather than replacing them. By moving beyond chat to more sophisticated patterns of human-AI collaboration, we're building interfaces that don't just respond to commands but truly understand and anticipate user needs.
We deliver this in a user interface that in some ways should feel familiar to other applications that you've used—it's just that the brain powering all of it is really what creates that user delight magical moment.
The journey is just beginning, but one thing is clear—the future of AI interfaces lies not in mimicking human conversation but in creating entirely new ways for humans and AI to work together.
The Chat Disappointment
I initially embraced the chatbot interface like everyone else in the design world. It seemed like this perfect marriage of AI capability and user familiarity - a simple text box where users could ask for anything they needed. When we began our work on an innovation management platform for medical researchers, it felt natural to center the experience around a conversational AI interface.
We built beautiful prototypes. We crafted detailed prompt engineering. We were confident we'd created something revolutionary.
And then we put it in front of actual users.
Chat was very confusing, and actually would dominate the entire experience so much so that people wouldn't look elsewhere on the screen. They were missing a lot of the context that was being created for them. The chat interface wasn't just underperforming—it was actively undermining the experience we wanted to create.
This is the story of how I discovered that the future of AI interfaces lies not in replicating human conversation, but in reimagining UI patterns that create true human-AI collaboration.
The Journey to Something Better
The initial stakeholder excitement about chat interfaces created its own momentum. There's something deeply compelling about the demo of a responsive AI chatbot answering questions with human-like fluency. This excitement led to our POC (proof of concept) being built around a chat-first approach.
But as we continued user testing, the evidence became impossible to ignore. Users weren't effectively accessing the key information and capabilities of the system through chat alone. They struggled to form effective queries and often missed important details that were presented in the chat stream.
We spent a lot of cycles helping our client understand that the POC, at least the user experience side of the POC, we needed to forget about if we wanted to make a good experience for the users. This was a pivotal moment—admitting that despite all the initial excitement, we needed to fundamentally rethink our approach.
Breaking free from the constraints of chat-only interfaces opened up an entirely new world of design possibilities. I began exploring how AI could enhance traditional UI patterns rather than replace them. What if, instead of forcing users to engage with an AI through conversation, we let the AI enhance the interfaces users already understood?
Emerging Patterns That Transformed the Experience
Dynamic Blocks: UI Elements That Respond to Context

Our breakthrough came in the form of what I call "dynamic blocks"—UI components that appear, populate, and adapt based on AI analysis of user needs and context.
For medical innovators uploading documents about their research, the system began analyzing the content and automatically structuring it according to the Stanford Bio Design framework. But rather than presenting this analysis as a chat message, we displayed it as a visual grid of information blocks, each representing a different aspect of the innovation.
The transformation was remarkable. Instead of users having to parse through chat messages to find relevant information, they could immediately see what the AI had understood and what it was missing. The interface became a living, breathing entity that evolved alongside the user's work.
One innovator with over a dozen patents and technologies already in hospital settings told me this thing is already allowing him to spend more time with his family. He actually has a deeper sense of peace, knowing that there's this tool that's doing active research and is aware of market changes in the cases that he's innovating in, and he doesn't have to use so much mental bandwidth to keep track of all of it.
By shifting from chat to dynamic UI elements, we'd created something that felt less like talking to a robot and more like working with an intelligent system that anticipated needs.
Governor Patterns: Building Trust Through Verification
A key insight I gained was understanding the importance of "governor patterns"—UI elements that give users control over AI-generated content. You can read an excellent overview of governor patterns on Shapeof.ai.
Rather than having the AI automatically populate information that might be incorrect or misaligned with the user's intent, we designed a review workflow. When new information blocks appeared, they were initially displayed at 70% opacity to indicate their provisional status. Users could review the content, make adjustments if needed, and then approve it—bringing the block to full opacity and marking it as verified.
This seemingly simple pattern had profound effects on user trust. By acknowledging the imperfect nature of AI and giving users the final say, we transformed AI from a black box into a collaborative tool. The governor pattern created what I'd describe as a human-in-the-loop feedback loop that maintained the user's sense of ownership while still leveraging AI capabilities.
Milestone Markers: Guiding Without Controlling
The third pattern that fundamentally transformed our interface was what I call "milestone markers"—visual indicators that help users understand their progress and suggest potential next steps.
In a traditional interface, the path forward is predetermined by the designer. In a chat interface, the path can feel completely unstructured. Milestone markers represent a middle ground—AI-generated suggestions that guide without restricting.
For our medical innovators, these markers highlighted potential gaps in their innovation documentation and suggested resources to address them. Rather than forcing users down a linear path, the AI analyzed their unique situation and offered personalized guidance.
The user gets a ton of visual affordance to sort of know what's left, what have I done. And it does deliver quite a bit of delight to the user coming in... This system is already telling you where the gaps exist in your idea and really what you need to work on to take it forward. And we do all of this without a chat interaction, without you needing to read through a ton of text or understand how to use AI.
The Designer's Challenge
Creating these new patterns wasn't without significant challenges. Perhaps the biggest was in prototyping and testing.
Traditional prototyping tools like Figma excel at creating static mockups of predetermined states. But how do you prototype an interface that adapts dynamically to each user? How do you test an experience that might be different for every person who uses it?
Each one is like a bit of a snowflake, and the only way that we're able to assess quality and reliability is to get in front of the customer with working software so that they can make that determination for themselves.
This required a fundamental shift in our design process. Rather than creating comprehensive mockups of every possible state, I focused on designing at the atomic level—creating flexible components that could be assembled in countless ways.
We created what I call design components using atomic level design thinking... starting with your smallest parts, packaging up those atomic elements into organisms, blowing those organisms out into templates, sharing those templates with the developers... and then the developers implementing it in a way that ultimately leads to like thousands of variations.
The result was a design system that could scale to accommodate the unpredictable nature of AI interactions while maintaining visual consistency and usability.
For designers accustomed to controlling every pixel, this was a profound shift. In a traditional design world, you would try to design for all of those variants because it was very deterministic. But in this new world, it's not so black and white, it's not so straightforward. It's really taking out all the context that it has and then serving up a user interface that's really personalized individualized for that end user.
Lessons for Designers Creating AI Interfaces
Through this journey of redesigning AI interfaces beyond chat, I've gained several key insights that can guide other designers working on similar challenges:
1. Recognize the limitations of chat-only interfaces
While chat is intuitive and requires minimal onboarding, it often struggles to effectively organize complex information or guide users through multifaceted tasks. Don't let the initial excitement of conversational AI blind you to its limitations.
2. Design for collaboration, not replacement
The most effective AI interfaces don't try to replace traditional UI elements—they enhance them. Think of AI as a collaborative partner that works alongside familiar interface patterns, not a replacement for them.
3. Build governor patterns into your design
Users need to feel in control, especially when working with AI systems. Design explicit approval mechanisms that acknowledge the provisional nature of AI-generated content and give users the final say.
4. Create systems, not screens
With AI-driven interfaces, you're no longer designing static screens—you're designing systems that can generate countless variations. Focus on creating robust design systems with clear rules rather than trying to anticipate every possible state.
5. Develop new prototyping approaches
Traditional prototyping methods fall short for AI interfaces. I wish there was a button in Figma where I could create an AI dynamic track and then dynamically populate the values to create endless variations of the same components. Since that doesn't exist yet, consider creating "scenario prototypes" that show a few realistic variations, then move quickly to working software for true validation.
6. Challenge stakeholder assumptions
The allure of chatbots can create unrealistic expectations among stakeholders. Be prepared to advocate for more effective approaches based on user research, even when it means pushing back against initial excitement.
A New Design Partnership
The future of UI design isn't about choosing between traditional interfaces and AI—it's about forging a new kind of partnership between humans and intelligent systems. The patterns I've explored—dynamic blocks, governor mechanisms, and milestone markers—represent early steps toward interfaces that combine AI capabilities with human-centered design principles.
For our medical innovators, this partnership has been transformative. As one user told me, the system has given him a deeper sense of peace, knowing that there's this tool that's doing active research and is aware of market changes. It's allowed him to focus on what matters most—both in his work and in his life.
As I continue exploring this new frontier of design, the goal remains the same: creating experiences that amplify human capabilities rather than replacing them. By moving beyond chat to more sophisticated patterns of human-AI collaboration, we're building interfaces that don't just respond to commands but truly understand and anticipate user needs.
We deliver this in a user interface that in some ways should feel familiar to other applications that you've used—it's just that the brain powering all of it is really what creates that user delight magical moment.
The journey is just beginning, but one thing is clear—the future of AI interfaces lies not in mimicking human conversation but in creating entirely new ways for humans and AI to work together.
The Chat Disappointment
I initially embraced the chatbot interface like everyone else in the design world. It seemed like this perfect marriage of AI capability and user familiarity - a simple text box where users could ask for anything they needed. When we began our work on an innovation management platform for medical researchers, it felt natural to center the experience around a conversational AI interface.
We built beautiful prototypes. We crafted detailed prompt engineering. We were confident we'd created something revolutionary.
And then we put it in front of actual users.
Chat was very confusing, and actually would dominate the entire experience so much so that people wouldn't look elsewhere on the screen. They were missing a lot of the context that was being created for them. The chat interface wasn't just underperforming—it was actively undermining the experience we wanted to create.
This is the story of how I discovered that the future of AI interfaces lies not in replicating human conversation, but in reimagining UI patterns that create true human-AI collaboration.
The Journey to Something Better
The initial stakeholder excitement about chat interfaces created its own momentum. There's something deeply compelling about the demo of a responsive AI chatbot answering questions with human-like fluency. This excitement led to our POC (proof of concept) being built around a chat-first approach.
But as we continued user testing, the evidence became impossible to ignore. Users weren't effectively accessing the key information and capabilities of the system through chat alone. They struggled to form effective queries and often missed important details that were presented in the chat stream.
We spent a lot of cycles helping our client understand that the POC, at least the user experience side of the POC, we needed to forget about if we wanted to make a good experience for the users. This was a pivotal moment—admitting that despite all the initial excitement, we needed to fundamentally rethink our approach.
Breaking free from the constraints of chat-only interfaces opened up an entirely new world of design possibilities. I began exploring how AI could enhance traditional UI patterns rather than replace them. What if, instead of forcing users to engage with an AI through conversation, we let the AI enhance the interfaces users already understood?
Emerging Patterns That Transformed the Experience
Dynamic Blocks: UI Elements That Respond to Context

Our breakthrough came in the form of what I call "dynamic blocks"—UI components that appear, populate, and adapt based on AI analysis of user needs and context.
For medical innovators uploading documents about their research, the system began analyzing the content and automatically structuring it according to the Stanford Bio Design framework. But rather than presenting this analysis as a chat message, we displayed it as a visual grid of information blocks, each representing a different aspect of the innovation.
The transformation was remarkable. Instead of users having to parse through chat messages to find relevant information, they could immediately see what the AI had understood and what it was missing. The interface became a living, breathing entity that evolved alongside the user's work.
One innovator with over a dozen patents and technologies already in hospital settings told me this thing is already allowing him to spend more time with his family. He actually has a deeper sense of peace, knowing that there's this tool that's doing active research and is aware of market changes in the cases that he's innovating in, and he doesn't have to use so much mental bandwidth to keep track of all of it.
By shifting from chat to dynamic UI elements, we'd created something that felt less like talking to a robot and more like working with an intelligent system that anticipated needs.
Governor Patterns: Building Trust Through Verification
A key insight I gained was understanding the importance of "governor patterns"—UI elements that give users control over AI-generated content. You can read an excellent overview of governor patterns on Shapeof.ai.
Rather than having the AI automatically populate information that might be incorrect or misaligned with the user's intent, we designed a review workflow. When new information blocks appeared, they were initially displayed at 70% opacity to indicate their provisional status. Users could review the content, make adjustments if needed, and then approve it—bringing the block to full opacity and marking it as verified.
This seemingly simple pattern had profound effects on user trust. By acknowledging the imperfect nature of AI and giving users the final say, we transformed AI from a black box into a collaborative tool. The governor pattern created what I'd describe as a human-in-the-loop feedback loop that maintained the user's sense of ownership while still leveraging AI capabilities.
Milestone Markers: Guiding Without Controlling
The third pattern that fundamentally transformed our interface was what I call "milestone markers"—visual indicators that help users understand their progress and suggest potential next steps.
In a traditional interface, the path forward is predetermined by the designer. In a chat interface, the path can feel completely unstructured. Milestone markers represent a middle ground—AI-generated suggestions that guide without restricting.
For our medical innovators, these markers highlighted potential gaps in their innovation documentation and suggested resources to address them. Rather than forcing users down a linear path, the AI analyzed their unique situation and offered personalized guidance.
The user gets a ton of visual affordance to sort of know what's left, what have I done. And it does deliver quite a bit of delight to the user coming in... This system is already telling you where the gaps exist in your idea and really what you need to work on to take it forward. And we do all of this without a chat interaction, without you needing to read through a ton of text or understand how to use AI.
The Designer's Challenge
Creating these new patterns wasn't without significant challenges. Perhaps the biggest was in prototyping and testing.
Traditional prototyping tools like Figma excel at creating static mockups of predetermined states. But how do you prototype an interface that adapts dynamically to each user? How do you test an experience that might be different for every person who uses it?
Each one is like a bit of a snowflake, and the only way that we're able to assess quality and reliability is to get in front of the customer with working software so that they can make that determination for themselves.
This required a fundamental shift in our design process. Rather than creating comprehensive mockups of every possible state, I focused on designing at the atomic level—creating flexible components that could be assembled in countless ways.
We created what I call design components using atomic level design thinking... starting with your smallest parts, packaging up those atomic elements into organisms, blowing those organisms out into templates, sharing those templates with the developers... and then the developers implementing it in a way that ultimately leads to like thousands of variations.
The result was a design system that could scale to accommodate the unpredictable nature of AI interactions while maintaining visual consistency and usability.
For designers accustomed to controlling every pixel, this was a profound shift. In a traditional design world, you would try to design for all of those variants because it was very deterministic. But in this new world, it's not so black and white, it's not so straightforward. It's really taking out all the context that it has and then serving up a user interface that's really personalized individualized for that end user.
Lessons for Designers Creating AI Interfaces
Through this journey of redesigning AI interfaces beyond chat, I've gained several key insights that can guide other designers working on similar challenges:
1. Recognize the limitations of chat-only interfaces
While chat is intuitive and requires minimal onboarding, it often struggles to effectively organize complex information or guide users through multifaceted tasks. Don't let the initial excitement of conversational AI blind you to its limitations.
2. Design for collaboration, not replacement
The most effective AI interfaces don't try to replace traditional UI elements—they enhance them. Think of AI as a collaborative partner that works alongside familiar interface patterns, not a replacement for them.
3. Build governor patterns into your design
Users need to feel in control, especially when working with AI systems. Design explicit approval mechanisms that acknowledge the provisional nature of AI-generated content and give users the final say.
4. Create systems, not screens
With AI-driven interfaces, you're no longer designing static screens—you're designing systems that can generate countless variations. Focus on creating robust design systems with clear rules rather than trying to anticipate every possible state.
5. Develop new prototyping approaches
Traditional prototyping methods fall short for AI interfaces. I wish there was a button in Figma where I could create an AI dynamic track and then dynamically populate the values to create endless variations of the same components. Since that doesn't exist yet, consider creating "scenario prototypes" that show a few realistic variations, then move quickly to working software for true validation.
6. Challenge stakeholder assumptions
The allure of chatbots can create unrealistic expectations among stakeholders. Be prepared to advocate for more effective approaches based on user research, even when it means pushing back against initial excitement.
A New Design Partnership
The future of UI design isn't about choosing between traditional interfaces and AI—it's about forging a new kind of partnership between humans and intelligent systems. The patterns I've explored—dynamic blocks, governor mechanisms, and milestone markers—represent early steps toward interfaces that combine AI capabilities with human-centered design principles.
For our medical innovators, this partnership has been transformative. As one user told me, the system has given him a deeper sense of peace, knowing that there's this tool that's doing active research and is aware of market changes. It's allowed him to focus on what matters most—both in his work and in his life.
As I continue exploring this new frontier of design, the goal remains the same: creating experiences that amplify human capabilities rather than replacing them. By moving beyond chat to more sophisticated patterns of human-AI collaboration, we're building interfaces that don't just respond to commands but truly understand and anticipate user needs.
We deliver this in a user interface that in some ways should feel familiar to other applications that you've used—it's just that the brain powering all of it is really what creates that user delight magical moment.
The journey is just beginning, but one thing is clear—the future of AI interfaces lies not in mimicking human conversation but in creating entirely new ways for humans and AI to work together.
James is a designer specializing in AI interfaces. This post is based on his experience designing AI interfaces for innovation management, with some details modified for clarity and confidentiality.